Prediction of Supraglacial Lakes Extent
and Depth in Greenland
"This proof-of-concept study demonstrates the feasibility of using deep learning for SGL monitoring, addressing data limitations and improving accuracy compared to traditional methods."
Background
Supraglacial lakes (SGLs) are an important parameter for understanding the current and future state of the Greenland Ice Sheet. While previous research has primarily focused on mapping SGL extent using optical and radar imagery, accurately determining SGL depth has been proven to be challenging due to the sparse temporal and spatial coverage of laser altimeters such as ICESat-2. Conventional methods for assessing SGLs have also been constrained by data availability and depth estimation accuracy. This case study explores a novel deep learning approach to predict SGL extent and depth using a combination of ICESat-2 data Sentinel-2 imagery.
The Problem
The Greenland Ice Sheet has experienced significant mass loss due to increased surface melt and glacier dynamics. This phenomenon poses critical implications, particularly regarding the rises in sea level. In the last 20 years, the contribution of supraglacial lakes (SGLs) to this mass loss has become more pronounced, necessitating improved observational methods for SGL extent and depth.
The Solution
A supervised deep learning model was developed to predict SGL extent and depth, leveraging the complementary strengths of ICESat-2 and Sentinel-2 data. ICESat-2 provided precise elevation measurements, while Sentinel-2 offered high-resolution optical imagery for enhanced spatial context. We used U-Nets, a specific deep learning architecture that was originally developed for medical imagery applications but has shown a high level of success for a range of image segmentation problems. The skip connections between each convolutional step of the encoder and its’ corresponding decoder allow contextual information to be considered before making a prediction.
In the case of SGL, contextual factors like floating ice, sediment, and shadows can complicate the spectral signatures captured in optical imagery, which may result in inaccurate depth estimations. U-Nets are trained to handle these complexities by considering the overall context of the image.
It is evident in this figure that the model can be trained on ground truth labels to produce a model which creates a prediction for both SGL extent and depth from only Sentinel-2 imagery.
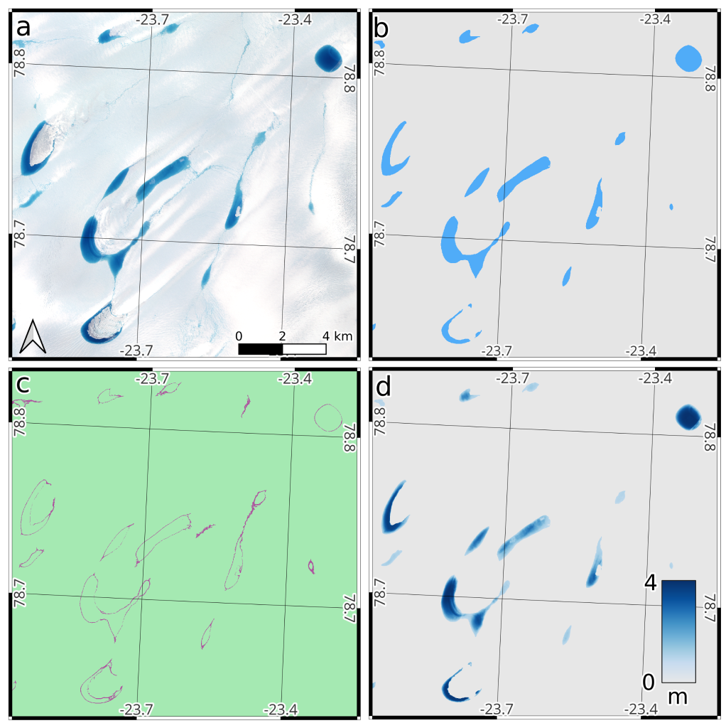